10. Evaluación de la retracción pulmonar mediante TCAR con la técnica de registro elástico para la monitorización de la fibrosis pulmonar idiopática
Abstract
Presentamos el comentario de un estudio retrospectivo que analiza el seguimiento y la evaluación de la fibrosis pulmonar idiopática mediante la tomografía computarizada de alta resolución (TCAR) y los test de función respiratoria. El objetivo de este estudio consiste en correlacionar el grado de retracción pulmonar mediante la técnica de registro elástico con TCAR y el deterioro funcional que se producen en la fibrosis pulmonar idiopática. Para ello, se realizó un registro elástico con dos TC para obtener mapas de deformación pulmonar. El registro elástico evaluó cuantitativamente la retracción pulmonar en la fibrosis pulmonar idiopática, correlacionándose con las pruebas de función pulmonar.
We present a review of a retrospective study that analyzes the evaluation and follow-up of idiopathic pulmonary fibrosis using high-resolution computed tomography (HRCT) and respiratory function tests. The aim of this study is to correlate the degree of lung shrinkage using elastic registration technique with HRCT and the functional impairment observed in idiopathic pulmonary fibrosis. Elastic registration was performed on HRCT to obtain lung shrinkage maps using a Jacobian determinant. Elastic registration quantitatively assessed lung shrinkage in idiopathic pulmonary fibrosis, correlating with pulmonary function tests.
Citas
Sun, X., Meng, X., Zhang, P., et al. (2022). Quantification of pulmonary vessel volumes on low-dose computed tomography in a healthy male Chinese population: the effects of aging and smoking. Quantitative Imaging in Medicine and Surgery, 12(1), 406–416. https://doi.org/10.21037/qims-21-160.
Chassagnon, G., Vakalopoulou, M., Régent, A., et al. (2021). Elastic registration-driven deep learning for longitudinal assessment of systemic sclerosis interstitial lung disease at CT. Radiology, 298(1), 189–198. https://doi.org/10.1148/radiol.2020200319.
Humphries, S., Yagihashi, K., Huckleberry, J., et al. (2017). Idiopathic pulmonary fibrosis: data-driven textural analysis of extent of fibrosis at baseline and 15-month follow-up. Radiology, 285(1), 270–278. https://doi.org/10.1148/radiol.2017161177.
Avants, B. B., Tustison, N. J., Stauffer, M., Song, G., Wu, B., & Gee, J. C. (2014). The Insight ToolKit image registration framework. Frontiers in Neuroinformatics, 8, 44. https://doi.org/10.3389/fninf.2014.00044.
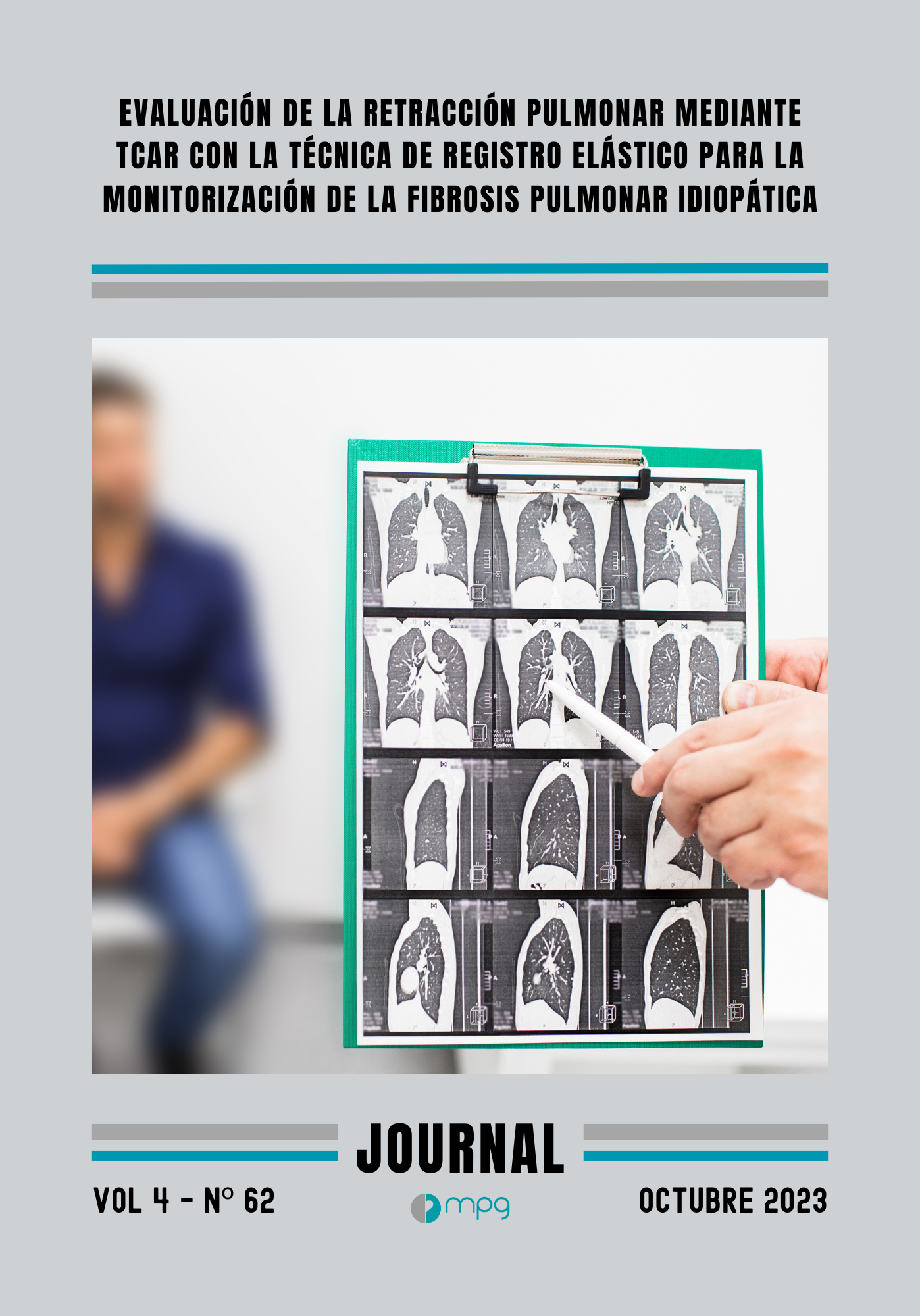
MPG Journal - Política de privacidad